About the seminar
This seminar aims to increase the links between the different laboratories in Saclay in the field of Applied Maths, Statistics and Machine Learning. The Seminar is organized every first Tuesday of the month with 2 presentations followed by a small refreshment. The localization of the seminar will change to accommodate the different labs.
Organization
Due to access restriction, you need to register for the seminar. A link is provided in the description and should also be sent with the seminar announcement. It will also help us organize for the food quantities. If you think you will come, please register! (even if you are unsure)
To not miss the next seminar, please subscribe to the announcement mailing list palaisien@inria.fr.
You can also add the calendar from the seminar to your own calendar (see below).
Next seminars
Scientific Committee
The program and the organization of this seminar is driven by a scientific committee composed of members of the different laboratories in Saclay. The members of the committee are currently:
- Arlot Sylvain (LMO, Université Paris-Sud)
- Blanchard Gilles (LMO, Université Paris-Sud)
- Brault Romain (ThereSIS, Thales)
- Brunel Victor-Emmanuel (CREST, ENSAE)
- Chiquet Julien (MIA, Agro Paris)
- Dieuleveut Aymeric (CMAP, Ecole polytechnique)
- Goude Yannig (R&D, EDF)
- Kalogeratos Argyris (CMLA, ENS Paris-Saclay)
- Kuhn Estelle (MaIAGE, Inra)
- Moreau Thomas (Parietal, Inria)
- Mozharovskyi Pavlo (LTCI, Telecom Paris)
- Pascal Frederic (L2S, CentraleSupelec)
- Quercini Gianluca (LRI, CentraleSupelec)
- Tami Myriam (MICS, CentraleSupelec)
Funding
This seminar is made possible with financial support of the ENSAE and DataIA.
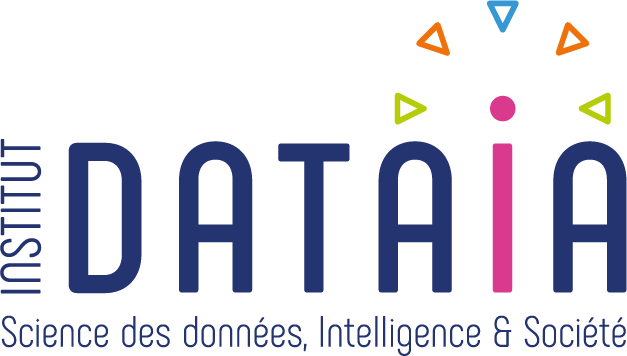
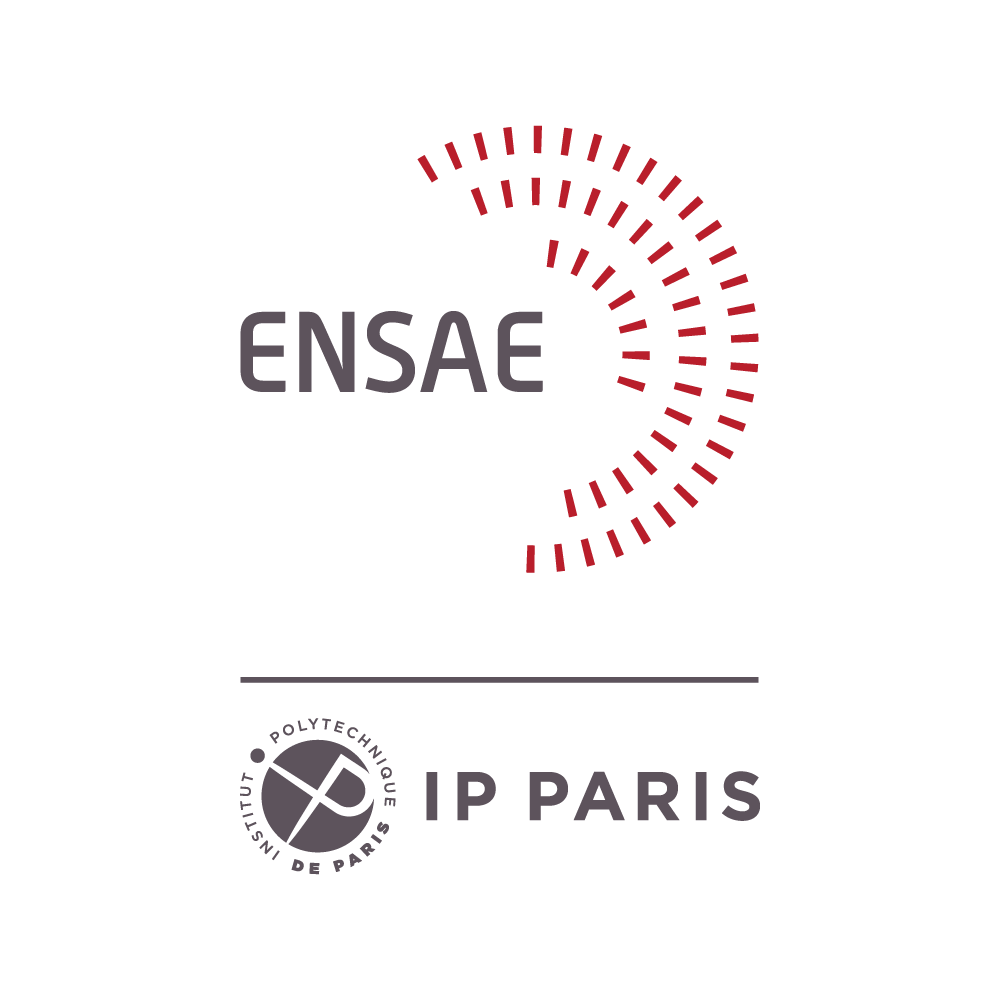